GPT-4: Commotion And Controversy
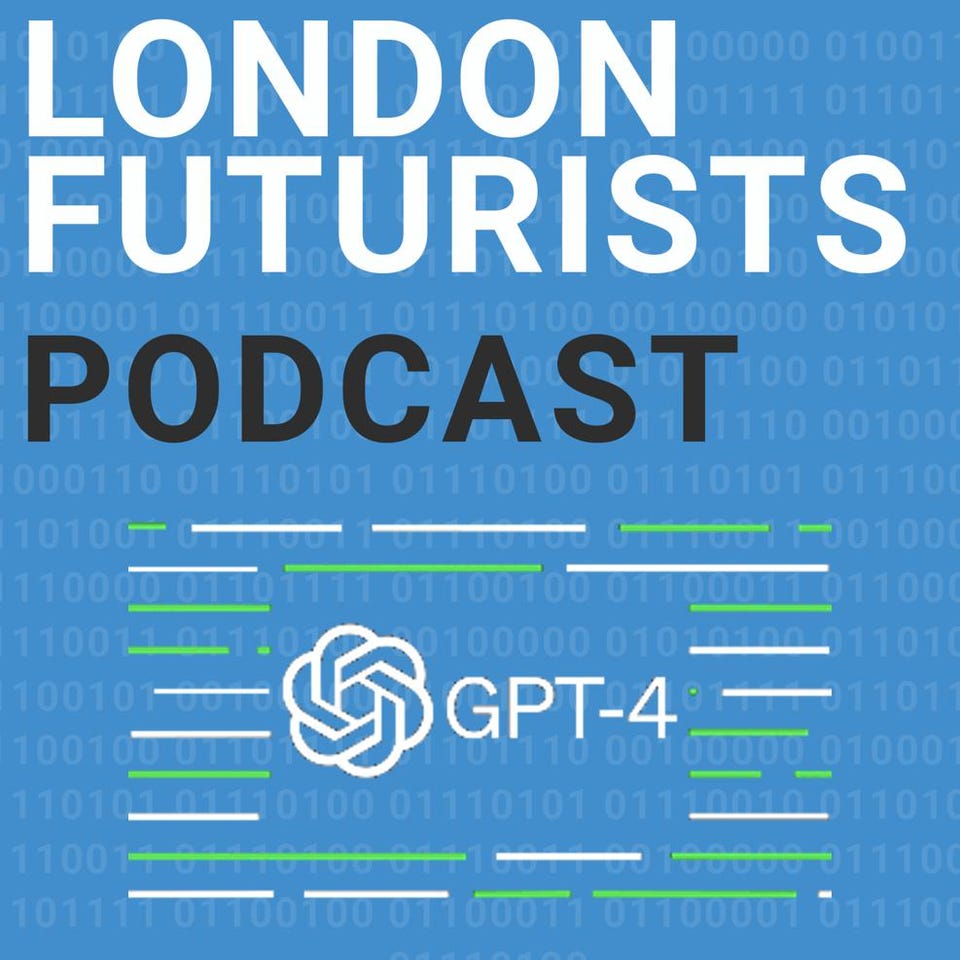
On the day that a London Futurists Podcast episode dedicated wholly to OpenAI’s GPT-4 system dropped, the Future of Life Institute published an open letter about the underlying technology. Signed by Stuart Russell, Max Tegmark, Elon Musk, Jaan Tallinn, and hundreds of other prominent AI researchers and commentators, the letter called for a pause in the development of the large language models like OpenAI’s GPT-4, and Google’s Bard.
It was surprising to see the name of Sam Altman, OpenAI’s CEO, on the list, and indeed it soon disappeared again. At the time of writing, there were no senior signatories from either of the two AGI labs, OpenAI and DeepMind, or from any of the AI-driven tech giants, Google, Meta, or Microsoft, Amazon or Apple. There was also no representation from the Chinese tech giants, Baidu, Alibaba, or Tencent.
Whatever you think of the letter’s prospects for success, and even the desirability of its objective, it was a powerful demonstration of the excitement and concern being generated in AI circles about GPT-4 and the other large language models. The excitement about GPT-4 is not overdone. The model is a significant advance on any previous natural language processing system.
The last time there was this much excitement about AI was in 2016, when DeepMind’s AlphaGo system beat Lee Sedol, the world’s best player of the board game Go. That achievement was the result of the Big Bang in AI which occurred four years before, in 2012. When a neural network developed by Geoff Hinton and colleagues won the ImageNet competition, it was the start of the deep learning revolution, and for the first time ever, AI began to make serious money. Interestingly, Hinton’s colleagues included Ilya Sutskever, who went on to help found OpenAI, and become its Chief Scientist.
GPT is the result of what may come to be called the second big bang in AI, which happened in 2017 when some Google researchers published a paper called “Attention is all you need”. This described a new type of deep learning called Transformers, which enable systems like Dall-E and Midjourney, that generate photorealistic images when prompted by short instructions in natural language. They also enable natural language systems like GPT-4.
This renewed public focus on AI is a good thing. The impact of AI on every aspect of life over the coming years and decades will be so profound that the more people are thinking about it in advance, the better. Even with GPT-4 hitting headlines, and obsessing many technophiles, AI still isn’t getting the wider public attention it really deserves. It still gets beaten by populist attacks on transgender people and other somewhat spurious stories, but we must be grateful for whatever progress we can get.
The operation of Transformers is often summarised as token prediction. They are trained on vast corpuses of text – all of Wikipedia, and millions of copyright-free books, for instance. They ingest this text and select tokens (words, or parts of words) to be “masked”, or hidden. Based on their model of how language works, they guess what the masked token is, and according to whether the guess was right or wrong, they adjust and update the model. By doing this billions of times, Transformers get really good at predicting the next word in a sentence. In order to avoid generating repetitive text, they make some arbitrary tweaks to the probabilities. When a system is tuned to make more tweaks, it is said to have a higher “temperature”.
Critically, this masking process does not require the training data to be labelled. The systems are engaged in self-supervised training. This is unlike the deep learning systems trained on massive datasets like ImageNet, where each image has been labelled by humans.
There is a human component in the training of transformers, though, which is Reinforcement Learning from Human Feedback, or RLHF. After the masking training is complete, the system’s responses to prompts are evaluated by humans for a period, and the evaluations are fed back into the system in order to minimise error and bias.
GPT-3, 3.5, 4
GPT stands for generative pre-trained transformer. GPT-3 was launched in November 2020 and boasted what was then an unheard-of number of 175 billion parameters (analogous to the synapses in a human brain). GPT-4 was released on 14 March 2023, and the number of parameters has not been disclosed. OpenAI has been criticised for reversing its policy of publishing as much as possible about its systems. It replies, not unreasonably, that if these models can cause harm in the wrong hands, it would be silly to make it easier for the bad guys to replicate them.
What is known is that the number of tokens that GPT-4 can handle – 32,000 – is much larger than the 4,100 that GPT-3 could manage. Among other things, this enables it to work with longer texts.
ChatGPT was a chatbot based on a halfway system, GPT-3.5. It was released in November 2022, and within a month it had 100m users, which was the fastest adoption rate of any app or platform.
OpenAI’s short and turbulent history
The story of OpenAI is as dramatic as its products are impressive. The company was formed in San Francisco in 2015 by Elon Musk, Sam Altman, and friends. They invested $1 billion of their own money to get the non-profit started. Musk stood down in 2018, and the reason given at the time was potential conflict of interest with his car company Tesla, which is also a significant developer of AI technology.
More recently there are reports that he left because he feared that OpenAI was failing to compete with the other leading AGI lab, DeepMind. (I call these two labs AGI labs because they are both explicitly targeting the development of artificial general intelligence, an AI with all the cognitive abilities of an adult human.) He offered to lead the company himself, and invest a further $1bn of his own money. When his leadership bid was declined, he left, taking the $1bn with him. OpenAI was unable to pay for the AI talent it needed, and its management decided it had to become, in part, a for-profit organisation.
Microsoft is very interested in GPT technology. It contributed $2bn before the launch of ChatGPT, and has agreed to invest a further $10bn since. OpenAI’s parent company is still a non-profit, and the returns to investors in the revenue-generating subsidiary are capped at 100x. Sam Altman, it turns out, has zero financial interest in the company. He doesn’t mind: he is already a rich man.
Musk has become a trenchant critic of OpenAI, especially on Twitter. Altman has expressed continued deep respect for his former business partner, but has also observed that he sometimes behaves like a jerk.
GPT-4’s advances
OpenAI’s latest system makes fewer mistakes than its predecessors – in the jargon, it hallucinates less. It is better at passing exams too. It passed the US bar exam with a score in the top 10% of candidates, whereas GPT-3 only managed the bottom 10%. This doesn’t tell us whether the system could actually be an effective lawyer, but it is impressive.
Unlike earlier systems, GPT-4 also seems to have learned simple maths. And it often appears to be doing something indistinguishable from reasoning. This was not expected from what are essentially pattern recognition systems. It has even led a group of Microsoft employees to publish an article claiming that GPT-4 shows the first sparks of AGI, although that has been characterised as hype.
Revised timelines and threat estimates
GPT-4 is impressive enough to be causing well-informed people to revise their timelines for the arrival of AGI, and of lasting widespread unemployment. Geoff Hinton, often called the godfather of deep learning, remarked in a recent interview that he used to think AGI was at least 20 years away, and very possibly 50. Now he thinks it might be less than 20 years. He also (for the first time, as far as I know) said that it is “not inconceivable” that advanced AI could cause human extinction.