How Advances In AI Are Shaping The Conversational Intelligence Market
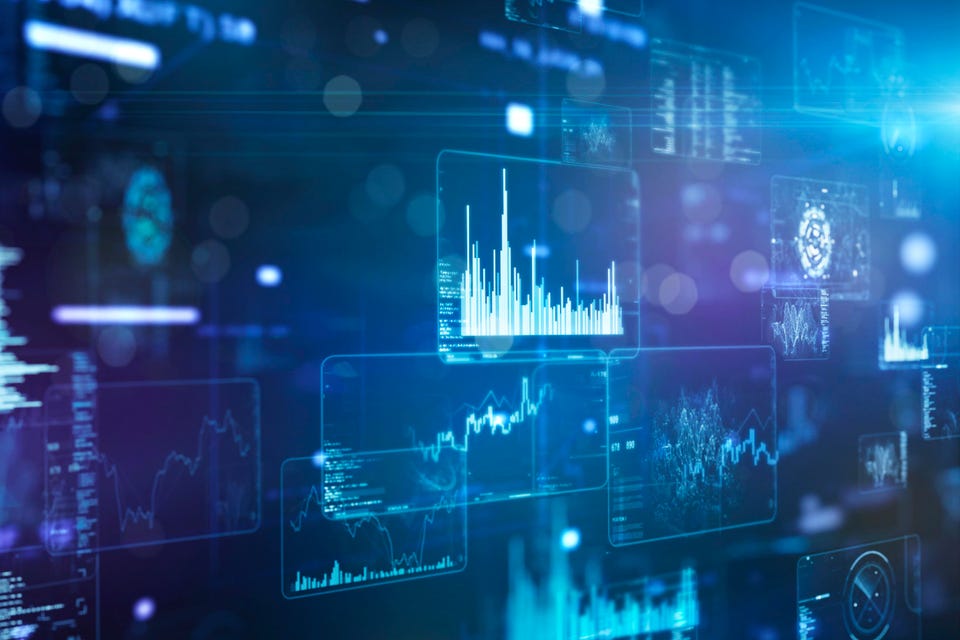
Conversational intelligence tools and artificial intelligence technology have always been tightly intertwined. The core challenge of conversational intelligence is to extract meaningful insights from large amounts of unstructured conversation data, and AI technology has long been the most effective way of doing so. Because of this, advances in AI capabilities result in new opportunities in the conversational intelligence space.
In the last few years especially, there has been an extraordinary rise in the capability and accuracy of AI systems to analyze voice, video and text data. Specifically concerning conversational intelligence, there are advances in three major areas that have created new possibilities.
1. Automated speech recognition.
2. Understanding and transformation of unstructured text.
3. Emotional analysis of video.
Automated speech recognition (ASR) refers to the technology used to transcribe spoken language into text. ASR relies on algorithms that analyze the acoustic characteristics of speech, such as pitch, tempo and phonetic content, to convert it into a digital format that can be processed by machines.
ASR is a critical part of conversational intelligence tools, as most natural language processing (NLP) models used in these tools are trained to work on plain text, and very few can work with raw audio data directly. This means that for almost every tool, the raw audio that gets inputted must be “translated” into machine-understandable text through ASR.
The accuracy of ASR systems has significantly improved in recent years, making them much more reliable. This strengthens a wide swath of conversational intelligence tools because one of the biggest challenges in applying NLP to audio conversations is getting accurate source data to work with from raw audio. Accurately transcribing spoken language can be difficult due to variations in accents, dialects and speaking styles.
These errors can cause NLP tasks to fail or produce incorrect results. For example, if the name “John” is incorrectly transcribed as the word “join,” an NLP system would incorrectly answer a question like “Which people did we mention in this conversation?”
However, recent advances in machine learning algorithms and speech recognition technologies have led to significant improvements in transcription quality, making it possible for machines to accurately transcribe and interpret human speech in real time. The word error rate of transcription providers has decreased by up to 6% in the last two years, and top new models like OpenAI’s Whisper can achieve word error rates of less than 10%.
By leveraging these improvements in transcription quality, conversational intelligence tools can more accurately understand and analyze human conversations, provide more relevant and personalized recommendations and deliver a more seamless and intuitive user experience.
Alongside the improvements in raw data from next-generation ASR, advances in artificial intelligence have also enabled us to understand the raw data at a much deeper level and perform much more advanced analysis and transformation of raw data.
Companies such as OpenAI, with their release of GPT-3 and now GPT-4, as well as ASR companies like AssemblyAI, Deepgram and Rev, have created many new avenues for extracting important information from unstructured text. Some of these types of analysis are:
AI algorithms can identify the overall sentiment of text data, whether it is positive, negative or neutral. This allows businesses to gauge customer sentiment about their products and services and adjust their strategies accordingly.
Topic Modeling
AI can identify the main topics and themes in a document or set of documents. This capability is useful for identifying trends and patterns in large volumes of text data.
Named Entity Recognition
AI can “identify and extract named entities, such as people, organizations, and locations” from text data. This capability is useful for categorizing and organizing text data.
Text Summarization
AI can extract the key points from large amounts of freeform text data.
Question Answering
AI can answer questions posed in natural language by extracting relevant information from text data. This capability is useful for chatbots and virtual assistants.
Overall, these new AI capabilities make it possible for conversational intelligence tools to deliver value in completely new ways. For example, companies like Supernormal, a customer of Recall.ai and Otter.ai, have already rolled out GPT-3 text summarizations to extract the key points from meetings and video conferences.
AI’s Impact On The Conversational Intelligence Market
While the two advances we previously discussed were text-related, advances in AI-related to video processing have also had a major impact on the conversational intelligence market. With the rise of remote work and the rapid increase in the popularity of video conferencing, video and image data have also become an increasingly important part of conversational intelligence.
This is because it provides rich visual information that can enhance the understanding and interpretation of spoken language. In addition to audio data, video data can capture important nonverbal cues such as facial expressions, body language and gestures, which can convey additional meaning and context that may not be conveyed through spoken language alone.
Since 2020, researchers have developed specialized neural networks for emotional analysis of video and images, such as WSCNet, which perform significantly better on emotional analysis. WSCNet achieved 70.07% accuracy on the large-scale FI dataset compared to the previous state-of-the-art VGG-16 model, which achieved 63.75% accuracy.
With greater accuracy, this class of analysis has become far more useful to conversational intelligence companies. By incorporating video data into conversational intelligence tools, these tools can provide a more accurate analysis of conversations. Top companies in the customer experience space like Recall.ai customer Voxpopme are incorporating visual sentiment analysis into their products, highlighting the growing importance of this kind of data.
Conclusion
Advances in artificial intelligence are one of the key drivers behind the evolution of conversational intelligence software. Because AI is key to extracting meaningful information from large amounts of unstructured text, audio or video, improvements in accuracy, performance or capability directly translate into conversational intelligence tools becoming more useful and valuable. The simultaneous advances in automated speech recognition, understanding of unstructured text and emotional analysis of video in the last few years have driven a rapid increase in the capabilities of many conversational intelligence products.