The Supremacy Of Biases In AI
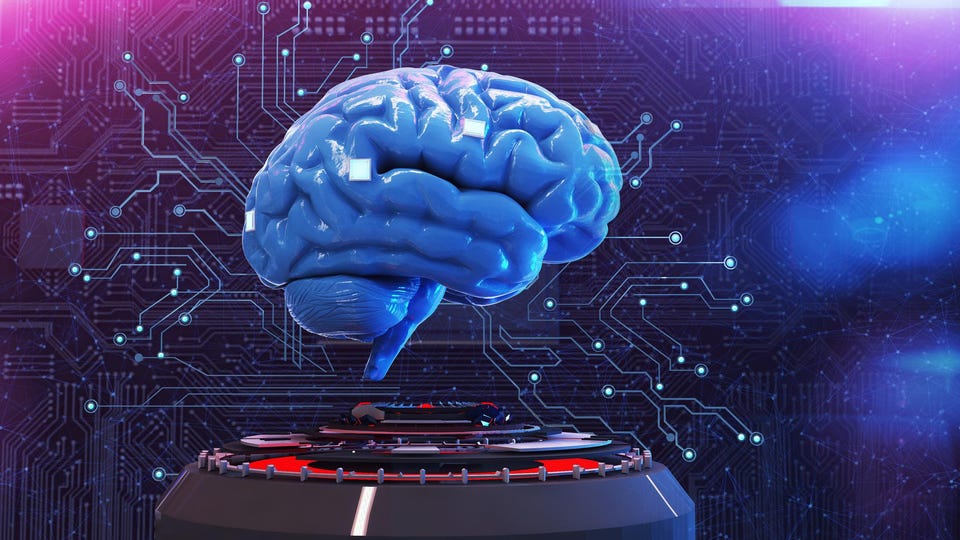
2022 might be remembered as the year that generative AI blasted on the scene, causing major waves in the digital community. With the onset of any new game-changing tech, in this case, the extremely powerful algorithms behind image and text generation systems such as ChatGPT and DALL-E, criticism and backlash are sure to follow. But I don’t believe that the problems and controversies associated with these algorithms should slow down their development.
The recent coverage on OpenAI’s outsourced content moderation is a fine example of how ambiguous the path forward might be. On the one hand, human oversight is inescapable; on the other hand, human moderators exposed to extreme content ought to get all the support necessary to conduct this brutal yet extremely fundamental part of the moderation process. Improving our standards when developing optimal versions for these algorithms is the best practice in all AI-involved scenarios.
Coincidentally (or not), 2022 was also the year I wrote extensively about the way that different cognitive biases and other processes influence our rationale, especially in the entire (both classic and deep neural networks-based) AI solutions. Incidentally (or not), the biases arising from these generative algorithms are the chief problem that needs to be tackled if we are to steer generative AI in a more productive direction.
Going forward, what can we draw from these biases? I want to take this opportunity, while 2023 is still fresh, to review the articles I’ve written covering these cognitive biases and how we can tackle these specifically when dealing with generative AI.
A short definition for confirmation bias is the cognitive path built into our subconscious according to our beliefs, which then redirects attention to arguments and pieces of evidence that reinforce this system of belief. This is one of the most pervasive biases in social media platforms. This is not an easy one to completely overcome: It takes constant practice and conscious effort to realize how they skew our rationale.
Survivorship bias reflects our tendency to choose only examples of success, the “survivors,” and to neglect all the negative examples of a certain case and what they would add to the dataset. I’m sure you’ve seen and heard about successful entrepreneurs, actors, athletes and all the stories behind them, but plenty of failed attempts are unknown for each success story. This example of bias is also quite widespread, and not just in social media, but in any other media since the dawn of the communication age.
The axiom that best defines false causality is that “correlation does not imply causation,” but even with this knowledge, it’s still common to make a strong association between two variables not necessarily related to one another.
Availability bias comes from the mental shortcut we use to make quick judgments, known as the “availability heuristic.” We tend to use the information most easily available to our reasoning, making it easier to miss the big picture; more often than not, you don’t have the full picture available to make your decision. This is because situations we observe or remember vividly produce quite an impact on our subconscious.
All the biases listed above emerge under specific cognitive conditions, and recognizing them gives us a huge advantage. But how can AI professionals effectively remove them from the decision-making process when working on an AI project?
Essentially, the answer to cognitive biases is outside the realm of one’s own cognitive processes, meaning that you can’t 100% trust how you conceive the problem and how you alone will figure it out. In practice, this means putting some distance between you and the problem at hand before deciding on a solution. Clearly, teamwork has crucial value here. Having a second, third or even fourth opinion will start to chop the biases from each individual and work to reach a common, objective viewpoint. The more diverse the team, the better, as the importance of diverse human oversight cannot be overstated.
The case of generative AI, however, presents some more complex challenges due to the tech’s grandiose objectives. To be as robust as possible, the algorithm needs a vast amount of training data—and this is where cognitive biases abound. Each different entry has the possibility to be profoundly biased. To perform mitigation on each of these would require an infinite amount of work. These extremely powerful tools require extremely powerful approaches in the case of biases, working on the data before the training or through some form of data filtering.
The case for pre-training mitigation is a simple one to cut bias on its roots. The AI model’s configuration is based on how the training is done and what data is used; these are all human choices, after all. Presenting the ethical dilemmas before training-based development begins is one of the safest ways forward, but this drastically reduces the speed at which a company can build such a tool. The use of free, unrestrained training data was what allowed the robustness of these algorithms in the first place.
What about mitigation through data filtering? There is a case for using technology advancements directly on bias issues and training the algorithm with filtered datasets. One recent example is related to the fairness of representation, one of the key problems to be solved. Researchers at Google are working on a tool called “Latent Space Smoothing for Individually Fair Representations” or LASSI. While notable and noble, making this kind of data-filtering solution effective on the ground level, however, is shaping up to be a tough endeavor.
Regardless of what solutions we come up with, it’s important not to let go of the ethical reasons for doing so. If human-made material for training and human moderation seems to be a vital part of the project, focus should be maintained on the processes deemed susceptible to human misconception. The tools being designed have amazing inhuman capabilities, but it’s the human component that will keep it suitable to our needs and mindful of our imperfections.