15 Key Mistakes To Avoid When Training AI Models
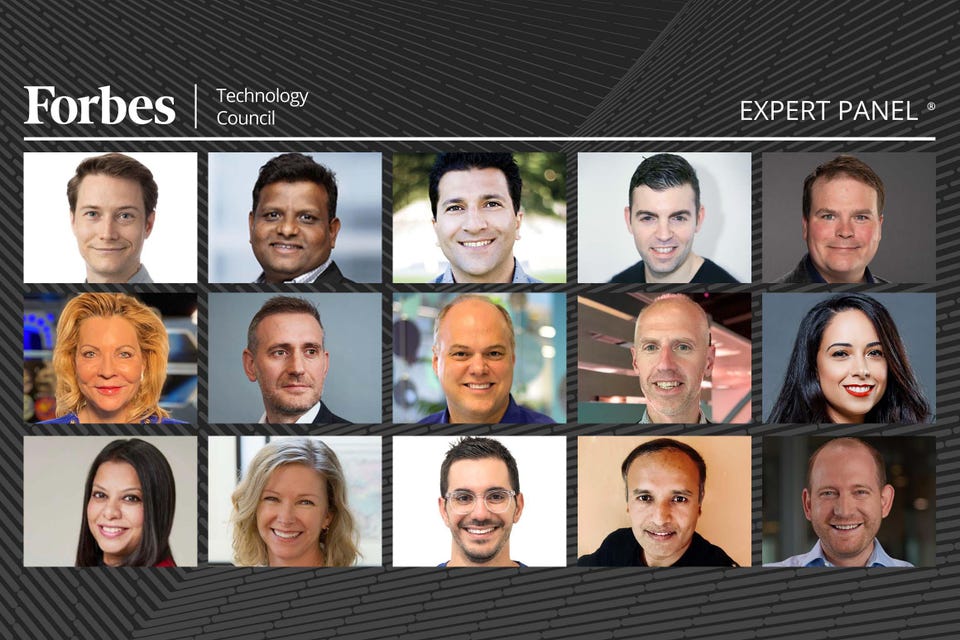
As artificial intelligence becomes increasingly prevalent in today’s world, the importance of carefully training AI models to perform complex tasks has become more critical. However, many organizations make common mistakes in the process of developing AI models, leading to suboptimal outcomes.
Some of these mistakes may include insufficient or low-quality data, not setting clear objectives, and failing to understand the limitations of the model. Below, members of Forbes Technology Council explore these and other common mistakes that organizations make when training AI models and provide tips on how to avoid them.
1. Not Monitoring Data Quality
I met the head of data at a self-driving car company. They paid to have image data labeled for them—“click images with bicycles,” for example. They trained the model, and performance tanked. As it turns out, their data had missing labels. Through basic monitoring, they could have caught it early, without retraining a big, expensive model on bad data. “Garbage in, garbage out,” as they say. – Kyle Kirwan, Bigeye
2. Not Using A Diverse Data Set
One key mistake organizations make in training AI models is failing to use a diverse set of data. This can lead to biased results. To avoid this, organizations should ensure the data used to train AI models is representative and diverse, reflecting a range of perspectives and experiences. Additionally, ongoing monitoring and testing can help detect and address any potential biases in the AI model. – Sunil Ranka, Predikly LLC
Forbes Technology Council is an invitation-only community for world-class CIOs, CTOs and technology executives. Do I qualify?
3. Not Having Enough Of The Right Kinds Of Data
One common mistake is not having enough qualitative, diverse and representative data to train a model. This can lead to poor performance and even harmful consequences. To avoid this, data should be chosen accurately to reflect the real-world population that the model will be making predictions about. – Fardad Zabetian, KUDO
4. Not Using Real Customer Data
AI companies tend to look for the “perfect data” for training and resort to synthetic or lab-generated references. In reality, data is contaminated and noise is everywhere, so the real challenge is to overcome it. I’ve found that most successful AI companies train models on real customer data and use their domain expertise to separate it from the noise. – Eliron Ekstein, Ravin AI Limited
5. Not Answering The Right Questions
Often, organizations don’t train their AI models to answer the right questions, or they don’t integrate them into their processes in a way that’s actionable. In the same way that generating business analytics often results in a dashboard that rarely gets looked at, trained AI models are only useful if they are predicting things that workers or customers care about. That can be hard to pinpoint. – Brian Jackson, Info-Tech Research Group
6. Having Biased Data
Biased data is a common problem companies have when training AI algorithms. The AI model will likely reinforce societal biases if the training data is biased. This can result in unfair or discriminating outcomes and significant repercussions. Companies must carefully pick and prepare training data and constantly monitor and evaluate AI models to eliminate bias. – Shelli Brunswick, Space Foundation
7. Not Making Judgments About ML Predictions
Machine learning models make predictions, not judgments. Outcomes can be wrong and look right, or be right and look wrong, and because machine learning is not easily interpretable, you cannot know the rationale behind the answer. Add this to the challenge of bias, and you can see why it is critical to consider how to apply a layer of judgment on top of any machine learning prediction. – James Duez, Rainbird Technologies
8. Overfitting The Model
There are many mistakes that newcomers make with this exciting technology. One is overfitting. Simply put, they overtrain the model on a particular set of inputs, and the model becomes narrow and brittle regarding any changes that don’t exactly mirror the training data. – Tod Loofbourrow, ViralGains
9. Failing To Architect End-To-End AI Solutions
Organizations typically fail to architect an end-to-end AI solution. They need to understand how experts make decisions today, what data is needed to improve predictions and how to collect and handle feedback for model management. They need to stop trying to see where AI technology can fit in their organization; instead, they need to start architecting AI like any other complex system. – Steven Gustafson, Noonum, Inc.
10. Not Curating And Balancing Training Data
Organizations can avoid a key mistake in AI model training by carefully curating and balancing their training data. Biased data can cause inaccurate or unfair predictions, so organizations should regularly evaluate their data for biases and mitigate them through techniques such as oversampling, data augmentation or bias removal. This leads to more ethical and effective AI models. – Imane Adel, Paymob
11. Neglecting To Define Objectives
Organizations frequently neglect to adequately define and validate their objectives when training AI models. Without specific goals, it can be challenging to judge an AI model’s effectiveness, which can result in subpar performance or unforeseen effects. – Neelima Mangal, Spectrum North
12. Not Including The Customer’s Voice
The biggest mistake is not including the voice of the customer. If you include a customer in AI training sessions, it will bring guaranteed insights that no one in your organization possesses. As an added benefit, including them can help make an important customer feel even more important. – Rhonda Dibachi, HeyScottie.com
13. Not Sanitizing The Data
Organizations frequently overlook properly curating and sanitizing the data used for training AI models, which is a critical error. Poor data quality can result in AI models that are biased, imprecise and unreliable. Negative effects, including poor judgments, missed chances and reputation loss, may occur from this. – David Bitton, DoorLoop
14. Not Ensuring Data Represents Good And Bad Behaviors
If AI models are trained on the wrong data, the predictions will also bake in those incorrect behaviors. When dealing with AI and ML applications for security and avoiding data breaches, ensuring that the data represents good and bad behaviors is essential. Data quality during feature mining—ensuring the right labels are in place for supervised learning—is important for organizations training AI models. – Supreeth Rao, Theom, Inc
15. Not Accounting For Data Shift And Semantic Shift
As an organization scales and moves into new domains, countries and business lines, the data that its models were trained on starts to shift based on the data that its users are currently inputting. The training of AI models needs to be a constant process, and a lot of attention needs to be paid to acquiring quality, representative data. – Isaac Heller, Trullion